
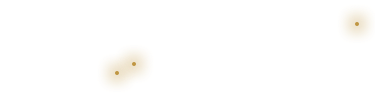
as seen on
Clean rooms are riding a wave of momentum as the ad industry looks for ways to use aggregated, anonymized data sets to predict audience identity. Yet, despite a catchy name, clean rooms aren’t necessarily as “clean” as they promise to be.
Though they are often being positioned as a magical space where the laws of privacy don’t apply, there are several dirty truths in the clean-room space.
If the industry is willing to shift its approach clean rooms can be valuable tools in the future of ad targeting.
“Clean room” is a misnomer
Perhaps the biggest issue with clean rooms is the lack of a common understanding of how they operate and the privacy issues they purport to address. This confusion enables some to exploit the gray areas.
Calling the product a “clean” room is an applaudable marketing feat, but matching a consumer’s personally identifiable information (PII) and converting it into device identifiable information (DII) should keep CMOs up at night – or at least raise an eyebrow.
It’s wishful thinking to believe PII goes into a clean room, up in the cloud, gets encoded and matched to another data set, then comes back in a privacy-safe format. Clean rooms can’t magically erase privacy laws. They can only push processing activities into a place enforcement hasn’t found yet.
Transparency, choice and data governance are the new standard
Basic privacy rules still apply in clean rooms: Marketers must secure the rights to use any data. But they must consider other questions as well.
What rights do consumers have with regard to data processing? If they have been informed their data is being processed, are they offered the ability to change their mind and opt out? How is the option to opt out communicated to other clean-room partners? Imagine how consumers would feel if they learned their PII is sitting in a clean room where others might have access to it.
Clean rooms have to have governance in place to ensure combining certain data types doesn’t violate the applicable privacy rules. There should be limits on the types of data that may be used in the clean room.
The cleanest method yields a better result
Assuming we follow applicable law and industry best practices, the concept of a clean room is solid. But a key shift needs to take place. There is a huge opportunity for the industry to abandon activating identity at the point of aggregation and instead embrace identity at the point of impression.
Working with this premise changes the requirements for clean rooms. Rather than taking PII and converting it to deterministically identifiable information, clean rooms would be the cleanest and most consumer-friendly if the actionable outputs of the comparative data sets were fully probabilistic.
The cleanest way to use clean room data is to only look at anonymized, aggregated analytics and insights, then use them to build out predictive audiences that combine comparative audience insights and contextual data. Done at the point of impression, this delivers a better, more privacy-friendly framework for targeting. It is cookieless, device-less and devoid of any deterministic or persistent identifiers.
The same basic concept remains: Two or more audiences are put together to best understand their intersection. The deliverable is an ID-free predictive audience that can be mapped against contextual data surrounding the impression itself versus a deterministic identifier.
Importantly, beyond being more privacy safe, this approach is better for advertisers. Predictive data sets can achieve far greater actionable scale across the entire open web.